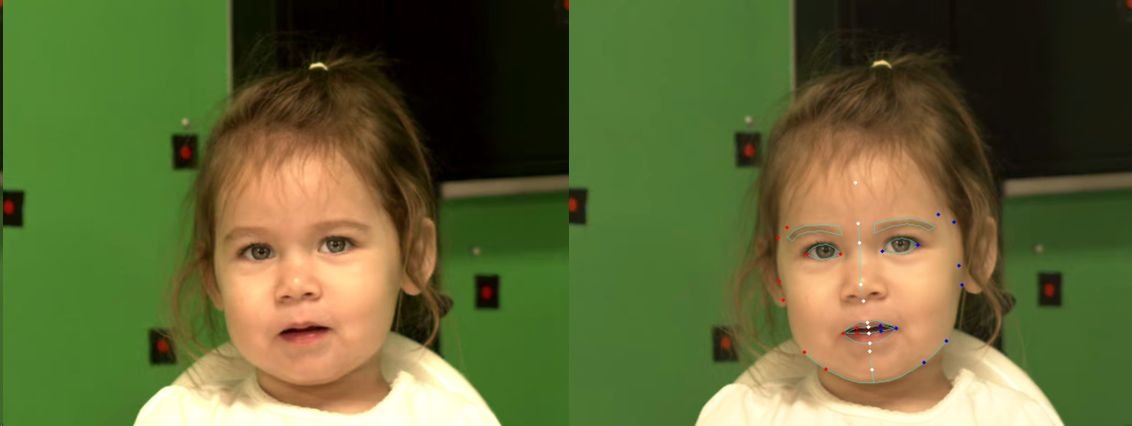
Speech Movement and Acoustic Analysis Tracking (SMAAT)
The Speech Movement and Acoustic Analysis Tracking (SMAAT) team is working to develop software for automatic speech movement and acoustic analysis. The software uses artificial intelligence and machine learning to support clinician’s diagnosis of a Speech Sound Disorder (SSD).
Our SMAAT software innovation will be a portable non-invasive web or stand-alone application designed to revolutionise the health services through improving the accuracy and (time) efficiency of differential diagnosis of SSDs in children. The availability of SMAAT could allow allied health professionals to determine the urgency of care (triage) for patients and could help alleviate the strain on the healthcare system. This would reduce waiting time for access to a speech-language pathologist, introduce a telehealth option and reduce the need for face-to-face contact, particularly beneficial for rural and remote populations. This platform will increase the accuracy of diagnosis, reduce administration time, lower financial cost, and provide timely access to personalised intervention.
Our research aims are as follows:
Development phase
Identify and derive distinct and repeatable articulatory, acoustic and phonetic features, of clinical interest, using audio-visual data.
Evaluation phase
Utilise computer vision and statistical learning approaches to create automatic scoring of features for clinical output.
External validation phase
Validate and trial a prototype for clinical implementation.
Background
Speech Sound Disorders (SSDs) encompass a range of difficulties in speech planning and production that affects as many as 20% of preschool children. A SSD not only puts a child at serious and immediate educational disadvantages, activity limitations, frustration, and isolation but also has a huge impact on a child's quality of life. Timely and accurate diagnosis is therefore vital to receiving the right treatment, and reducing the impact of the disorder. However, due to several significant barriers, it is difficult to determine the presence or absence of a SSD and to differentially diagnose a SSD. These barriers include insufficient time and clinical resources, limited clinical expertise in remote areas, and inefficient and variable assessment procedures with compromised accuracy.